
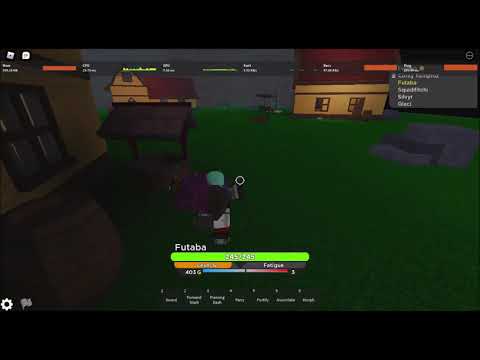
Comparison matrices have been shown over the experimental results of the various categories of topic modeling. We also covered the implementation and evaluation techniques for topic models in brief. Besides, the research work for topic modeling in a distributed environment, topic visualization approaches also have been explored. We first introduce the preliminaries of the topic modeling techniques and review its extensions and variations, such as topic modeling over various domains, hierarchical topic modeling, word embedded topic models, and topic models in multilingual perspectives. In this work, we study the background and advancement of topic modeling techniques. Probabilistic Topic Modeling discovers and explains the enormous collection of documents by reducing them in a topical subspace. A computational tool is extremely needed to understand such a gigantic pool of text. We are not able to deal with a mammoth text corpus without summarizing them into a relatively small subset. Community Topic is able to find this hierarchy more quickly and allows for on-demand sub- and super-topic discovery, facilitating corpus exploration by researchers. We compare the topic hierarchies discovered by Community Topic to those produced by two probabilistic graphical topic models and find that Community Topic uncovers a topic hierarchy with a more coherent structure and a tighter relationship between parent and child topics. Similarly, super-topics can by found by mining the network of topic hyper-nodes. The fractal structure of networks provides a natural topic hierarchy where sub-topics can be found by iteratively mining the sub-graph formed by a single topic. We develop a novel topic modelling algorithm, Community Topic, that mines communities from word co-occurrence networks to produce topics. However, topics naturally exist in a hierarchy with larger, more general super-topics and smaller, more specific sub-topics. The most popular topic modelling algorithm, Latent Dirichlet Allocation, produces a simple set of topics.
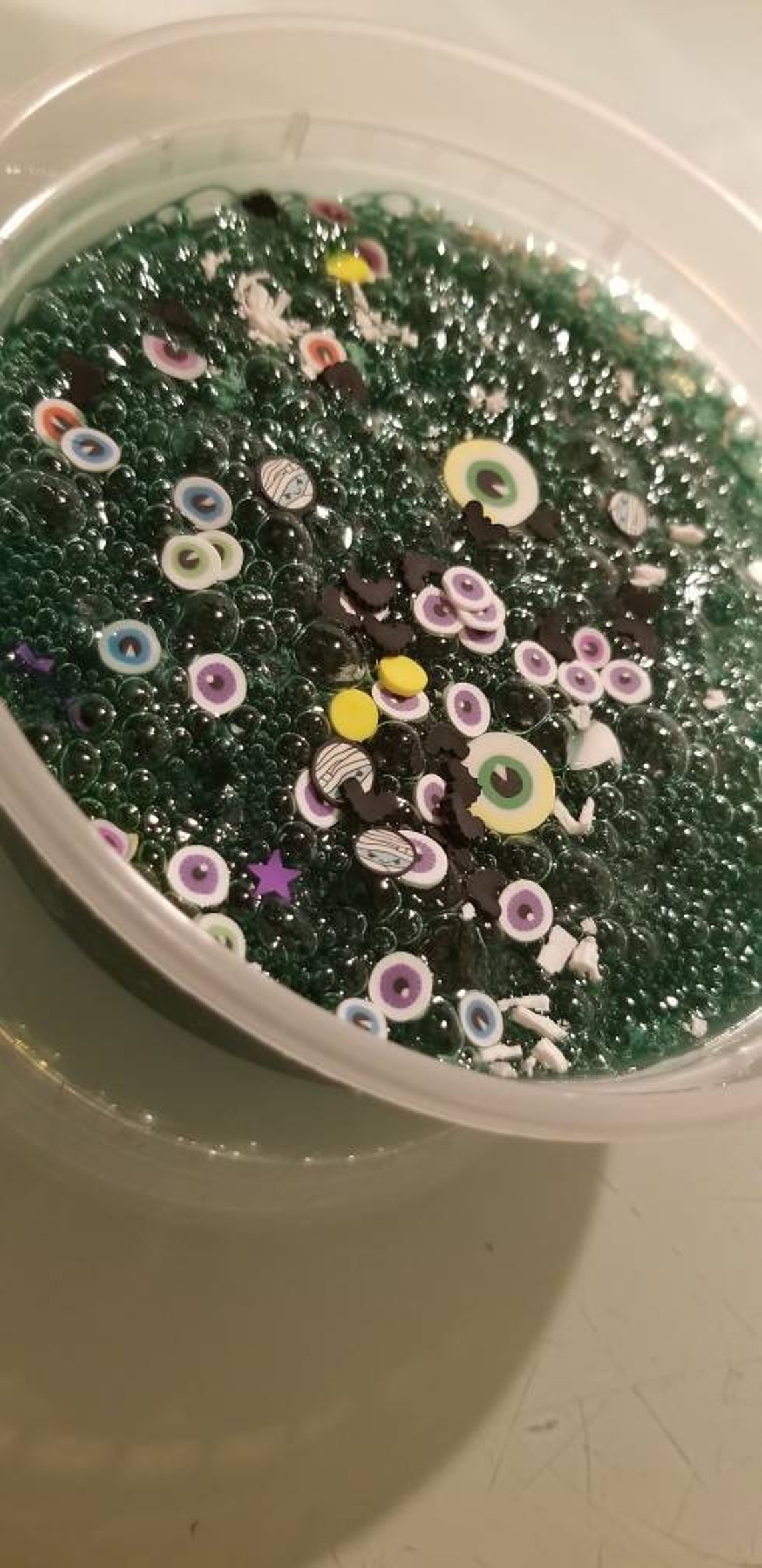
Then, we illustrate the evaluation metrics used to assess the quality of the generated hierarchical structure in extracting an ideal tree. In this paper, we present sentiment analysis models that analyzing people feelings and opinions in the short text such as tweets and instant messages. However, some methods produce an improper result when applied to short text due to text briefness and sparsity. Different sentiment analysis approaches have been proposed to understand the sentiments and opinions expressed by the individuals in the text. Sentiment analysis methods appear to analyze sentiments and opinions of people through what they write on social networking sites. So, it is necessary to make an intelligent system that automatically analyzes a great amount of data. It is challenging to analyze such a huge amount of information that causes time-consuming. Recently, the remarkable growth of Internet technology, particularly on social media networking sites, enables gathering data for analyzing and gaining insights. The results show that rCRP discovers a hierarchy in which the topics become more specialized toward the leaves, and topics in the immediate family exhibit more affinity than topics beyond the immediate family. We suggest two metrics that quantify the characteristics of a topic hierarchy to compare the discovered topic hierarchies of rCRP and nCRP. We compare the predictive power of rCRP with LDA, HDP, and nested Chinese restaurant process (nCRP) using heldout likelihood to show that rCRP outperforms the others. We apply rCRP to a corpus of New York Times articles, a dataset of MovieLens ratings, and a set of Wikipedia articles and show the discovered topic hierarchies. Unlike previous models for discovering topic hierarchies, rCRP allows the documents to be generated from a mixture over the entire set of topics in the hierarchy. We introduce the recursive Chinese restaurant process (rCRP) and a nonparametric topic model with rCRP as a prior for discovering a hierarchical topic structure with unbounded depth and width. While they are simple and popular, a major shortcoming of LDA and HDP is that they do not organize the topics into a hierarchical structure which is naturally found in many datasets. Topic models such as latent Dirichlet allocation (LDA) and hierarchical Dirichlet processes (HDP) are simple solutions to discover topics from a set of unannotated documents.
